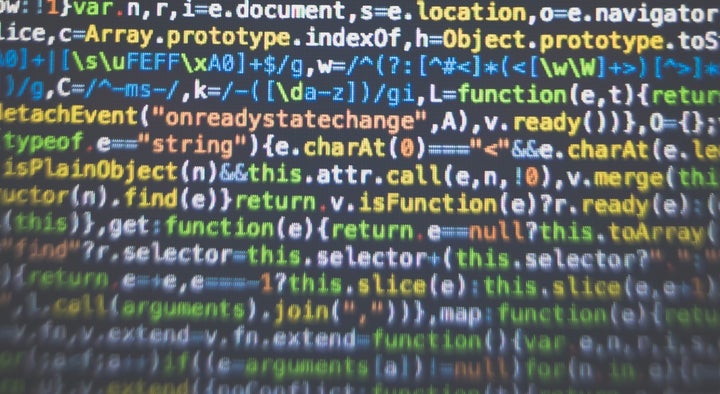
Big data algorithms have become trusted information shortcuts akin to maps.
There is more than just a passing methodological debate at stake in the question over what went wrong in the predictive models that were so far off for the recent U.S. presidential election. The capacity of contemporary elections to represent the will of the people is also at stake. To demonstrate why, begin by thinking of a map – perhaps the New York City subway map. Likely, you trust what you are looking at. Most of us do. Maps hang on classroom walls and in subway stations to help us find our way in a physical terrain too large and complex for any individual to observe first hand. Because modern societies need them to function, we are trained through societal norms to trust maps. As a result, maps are an especially powerful tool for shaping our perceptions of reality.
Despite our inherent trust, though, geographers like Mark Monmonier and Dennis Wood point out that maps lie to us all the time. Take the New York City subway map. It shows a highly distorted version of the city’s geography where Manhattan is made to look much larger than it actually is relative to the other boroughs in order to show all of the lines and stations clearly. This map effectively conveys information about the subways, but it also radically distorts millions of New Yorkers’ perceptions about the geography of their city. Likely this is a benign distortion, but you never know where such a misperception will make a difference.
Now think of the politics page on the website for any major media outlet during the run-up to the most recent presidential election – perhaps the New York Times or FiveThiryEight. If you were like me, you obsessively checked the large number and percent sign next each of the candidates’ names. These were the odds of winning, but not based on quixotic prognostications. Rather these odds were based on algorithms designed by predictive analytics specialists to display a political terrain that is too large and complex for us to observe on our own. Because no voter can be fully informed, political scientists like Larry Bartels have shown that modern democratic society needs such information shortcuts. So, as with maps, we are trained through societal norms to trust the models.
Especially since the predictions are carried everywhere by social media, analytic models have become powerful information shortcuts in American politics with intense capacity to shape our perceptions of reality. The algorithms also lie to us. We do not have a good understanding of the full range of distortions that are introduced, but we know that predictive models simplify political reality and collective behavior. Here the distortions that result can be far less benign than a misshapen vision of Manhattan. One obvious example of the effect seems to have been made clear by the last US presidential election.
Think, for example, of the undecided voters who did not fully support either candidate but preferred Hillary Clinton over Donald Trump. They saw between a 70 and 95 percent chance of Hillary Clinton winning based on most models. As a consequence, they might have reasonably assumed that the country had already chosen Hillary Clinton…few people on either side saw it differently. Thus the undecided voter felt more liberated than would otherwise have been the case to vote for a third party candidate or to not vote at all. We certainly saw evidence of this from the relatively low turnouts in cities and high third party numbers in some swing states.
If such a circumstance arose for a substantial number of voters, then the predictive analytic models affected the outcomes of the election in ways that were not accounted for in the models. In short, the model affected the model. This type of self-generated noise is pushed aside in predictive analytics in order to deliver an unambiguous signal to readers. In this way, the output of a predictive analytic algorithm lies to us in the same sense that the New York City subway map lies—it alters the content of the analysis in order to effectively deliver the message. This time around, the marginal shift in voter behavior that resulted from the distorted perception of political reality may have made a difference in the outcome for the entire electorate. Given this potential, we do well to remember that to predict is to commit a political act. Thus, no model can really capture political reality. We still have to gauge that for ourselves.